Data Science vs. Data Analytics: Two Sides of a Coin
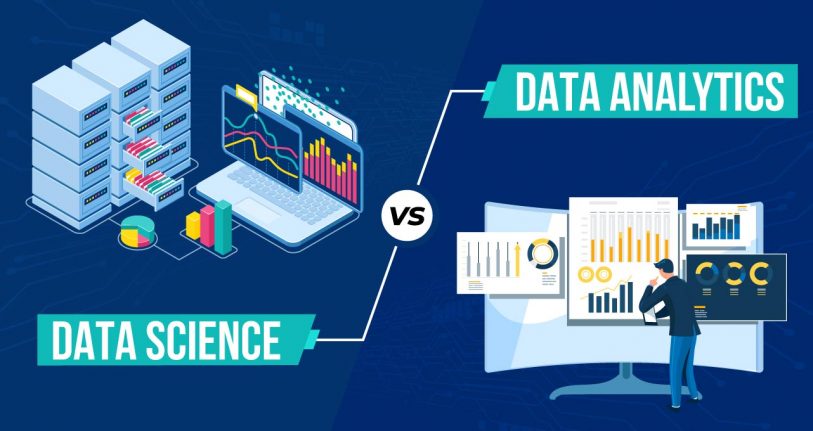
Regardless of the industry and organization size, companies are leveraging big data, to efficiently implement Data Science and Data Analytics in some way in their organizations. It also helps them improve their capabilities and avoid the risk of being left behind. With the boom of big data, the terminologies of “data science” and “data analysis” are tossed around without understanding the proper definition.
While they may sound the same, there are a lot of differences between the two. But before understanding a comparison between these two terms, let us understand these terms individually. We hope this blog will become a quintessential guide and help you understand these topics from scratch.
What is Data Science?
Data science is the application of machine learning algorithms to different types of structured or unstructured data. It uses the business or domain knowledge behind a given task and then unearthing some unseen insights or predicts something. In simpler terms, it can be said that data science is a blend of algorithms, tools, and machine learning principles, used to extract hidden information from raw unclassified data. Data Science focuses more on combining programming, statistics, data, and the domain
What is Data Analytics?
The process of exploring and analyzing big data to identify an unseen trend and discover correlation among the data to deliver a valuable insight is known as data analytics. By putting these insights into action, an organization can create personalized customer experiences, optimize the operations or increase employee productivity. Data Analytics focuses more on visualization, data, and the domain.
Data Science vs. Data Analytics
To explain the difference in a layman’s language – data science is focused more on the discovery of the insights from the data or predicting some future event using a combination of programming, statistics, domain knowledge. Whereas data analytics is more focused on uncovering or seeking insights from the existing data that you have using data visualization tools and domain knowledge. Data analytics focuses more on historical data while data science focuses more on machine learning and predictive modeling.
Another major difference between these two disciplines is how they are applied. Data science-primarily uses programming and machine learning whereas Data Analytics uses BI tools like Tableau, Metabase, and PowerBI. For example, data science can be used to create search engines through the use of algorithms to provide users with better-catered responses. But an application of Data analysis with respect to search engines can study how popular a few search terms are and what is the most searched item or topic via a given search engine.
Uses of Data Science & Data Analytics in E-Commerce Industry
These disciplines have made an important place in the e-commerce industry. From cross-selling to purchase predictions, e-commerce organizations use big data to make their decisions. Companies also use purchase data to create psychological portraits of a customer to market products to them and use it to drive customer loyalty and thereby more revenues.
Application of data science in e-commerce:
-
Recommendation Engine:
They are the most important tool in retail e-commerce. By providing recommendations, retailers increase their sales and dictate trends. The recommendation engines are made up of complex machine learning and deep learning algorithms designed in a way to keep the track record of every customer’s behavior and analyze their consumption pattern. It involves a great deal of data filtering and reading and all this happens via a machine learning model.
-
Price Optimization:
The most important task for a retailer/manufacturer is selling the product at the right price. The price not only includes the costs to make the product but also takes into account the ability of a customer to pay for that product while keeping in mind competitor prices as well to drive profits. All this is calculated with the help of machine learning algorithms. The algorithm analyses several parameters from the data like the flexibility of prices, location of the customer, the buying attitude of an individual customer, and competitor pricing.
-
Inventory Management:
The retailers should be able to stock the right goods in the right quantities in order to provide them to the customer when there is demand for that product. To achieve this, the stock and supply chains are analyzed thoroughly. Algorithms analyze the gaps between the demand and supply in great detail and detect patterns and correlations among purchases. By analyzing these data retailers/manufacturers come up with a strategy to increase sales, confirm timely delivery and manage the inventory stock.
Application of data analytics in e-commerce:
-
Customer Sentiment Analysis:
This has always been around the business for a long time, which is now simplified and automated with machine learning. This also saves a lot of time and gives accurate results. Currently, social forums are the most easily available tool for an analyst to perform customer sentiment analysis. Algorithms use natural language processing to identify words bearing a negative or positive attitude of the customer towards the brand. This feedback helps the business improve their product and services to meet consumer needs.
-
Measure the Marketing Effectiveness:
Data analytics can assist e-commerce companies in measuring how successful the marketing campaigns are, and also in improving decision-making, gaining more omnichannel traction, and informing holistic marketing programs.
-
Accurate Performance Tracking:
Ecommerce analytics gives the power to get a better understanding of how the business is performing now and how it is likely to perform in the near future. This analysis can be used to make informed decisions about everything from hiring goals and sales goals. It can also be used in making sure that the right products are accessible at the right time so that the customers’ expectations are met.
-
Better Supply Chain Management:
The problem that baffles most of the e-commerce companies out there is managing the supply chain effectively and hereby giving an optimal customer experience. Using data analytics, restocking and supply chain management can be improved drastically.
Summarizing
Even though these two sound the same, they vary at the very basic level. The end goal, tools being used, and the purpose of data are different in both cases. Data Analytics is a part of Data Science. Data Analytics deals with structured data to solve tangible business problems with help of programming languages like SQL, R, or python, data visualization software, or statistical analysis. Data Science deals more with unstructured data, using more advanced data techniques to predict the future. It also includes automating the machine learning algorithms to design predictive modeling processes that can handle both structured and unstructured data.
In the case of data science, the techniques are used to clean, process, and interpret data to extract insights from it. Advanced data modeling processes designed using prototypes, ML algorithms, and predictive models for custom analysis. On the other hand, data analytics examines the data set to identify the trends and draw conclusions from a large volume of collected data, organizing and analyzing it to identify a relevant pattern. Thus, we can say that data analysts transform complex insights into business-savvy language that can be understood by all decision-making members.
To sum it up, data science goes a step ahead of data analytics due to the predictive element. The key point to consider when employing a solution is whether you’re looking to make predictions based on data or to summarize/visualize big data sets. The former uses data science, the latter is a job for data analysis algorithms.